Data Quality Hub
Customer Data at the highest level
Improve the quality of your data with the Data Quality Hub. You ensure data quality right from the input stage, minimize errors in the overall database and create a usable database. This enables you to manage the quality of your customer data precisely and efficiently.
Optimize your customer data along the data quality lifecycle
To get the best out of your data, you need to understand, maintain, protect and monitor it. And you need to do this throughout the entire data quality lifecycle. For these tasks, we offer you a combination of powerful software and experienced consultants.
The Uniserv Data Quality Hub meets your requirements precisely. This ensures that your data always meets the requirements of your business.
Read the success story to find out how we mastered the challenges of data quality together with R+V Versicherung.
What does the Data Quality Hub do?
The Data Quality Hub offers a unique combination of flexibility, efficiency and user-friendliness. Unlike other solutions, it enables the rapid implementation of all desired data quality functions. This includes all applications that collect or change data from customers or interested parties, without high integration or development costs. Its system neutrality and the ability to add functions at the touch of a button make the Data Quality Hub a lean, targeted solution. Data stewards can work directly in the Hub interface if required.
Data Quality Hub Functions
Measure data quality - 5 tips for more transparency
When it comes to the quality of your own data, there is often nothing more than a vague gut feeling. So how do you make it all measurable?
Read the Uniserv business whitepaper 'Measurable data quality' to find out how you can switch from gut feeling to transparency when it comes to data quality and put it to the test.
Good data for your business processes
The importance of data quality for your company
Prospect and customer data is at the heart of companies. They are often not treated as such. They are inaccurate, inconsistent and out of date, scattered across different systems and often can no longer be easily merged. However, specialist departments need accurate, trustworthy and relevant data, for example:
With the Data Quality Hub you get:
- Relevant and valid prospect and customer data in one or more of your systems
- The ability to create a Unique Customer ID and a comprehensive 360-degree view
- Fast implementation and reduced development effort
- The flexibility to optimize and expand your data quality at any time and across systems
The Data Quality Hub brings you company-wide benefits:
- Data quality right from the start: Avoid errors as soon as you enter or change data with first-time-right data quality.
- Keep data smart and up-to-date: With the optionally selectable automatic functions for anti aging, data remains usable at all times.
- Enrich data easily: Different data enhancement functions are available depending on business requirements.
- Manage data efficiently: Centralize and maintain data across different systems.
- Automate: Reduce manual tasks and speed up business processes with automated data quality processes.
- Scale: Stay flexible to keep pace with growing data volumes and new business requirements.
- Save costs and time: Reduce manual corrections to data and conserve your resources with more efficient processes.
- Sound basis for decision-making: Reliable data allows you to improve your decisions and decision-making based on data.
- Meet compliance requirements: Comply with legal regulations and data protection provisions. This reduces risks and increases confidence in your own data.
- Optimize processes: Improve internal processes with high-quality data.
- Increase customer satisfaction: Provide personalized and accurate information for your customers and prospects. Improve customer satisfaction through personalized interactions.
Implement the Data Quality Hub quickly and easily
The Data Quality Hub integrates seamlessly into your existing IT infrastructure. It communicates with various systems via standardized interfaces. It checks and cleanses data in near real time or in batch, recognizes duplicates and enables the central management of data quality rules. Errors are not only detected, but also largely corrected automatically thanks to error-tolerant AI. This reduces the workload for data stewards. Functions, rules and interfaces can be individually configured to optimally meet the specific needs and processes of your company.
- The Data Quality Hub has a modular structure and can be flexibly adapted to different or growing requirements. New functions and systems can be integrated at any time without the need for extensive development projects in the application systems. This ensures long-term investment security.
- Thanks to its system-neutral architecture, the Data Quality Hub can be integrated into almost any IT environment. It supports common application systems such as Salesforce, MS Dynamics, SAP in the cloud or on-premises and even applications on z/OS mainframe systems. Integration takes place via standardized interfaces.
- Thanks to preconfigured modules and standardized interfaces, the Data Quality Hub can be implemented very quickly. You can be using the Data Quality Hub productively in no time at all.
Let us do it for you:
- Managed Service: If you prefer the Data Quality Hub to be operated in the Uniserv Cloud, we will take care of the entire managed service so that you no longer have to worry about operations.
- Application Management: If you prefer to operate the Data Quality Hub on-premises or in your private cloud, we will take over the application management for the Data Quality Hub.
Why Uniserv?
Data quality from the customer data expert
Why is it sensible to address the issue of data quality for customer and prospect data with Uniserv? Quite simply, you get the best quality from us. That's our promise!
- Data quality solutions from a single source.
- Practice-orientated expertise of our consultants.
- Flexible scalability of solutions and functions.
- Runs on all common platforms.
- Flexible scalability of solutions and functions.
The four levels of data quality
We will show you four steps that must be taken into account when it comes to the quality of the data so that it ultimately becomes a real asset for your company. Only the best for your data.
Download the Uniserv Business Whitepaper now.
Top companies put their trust in Uniserv
FAQs about data quality
In discussions with our customers and employees from the various departments, we are repeatedly told about similar symptoms of poor data quality. From the symptoms, clues for concrete data quality initiatives can be derived, resulting in initial indications for the creation of the catalog of measures:
- Customers and business partners appear more than once in the system
- Contact persons are not up-to-date
- High return rate for mailing campaigns due to incorrect or incomplete addresses
- Customer complaints about multiple deliveries of the same advertising mailings
- Low response rates for marketing campaigns
- Incorrect letter salutations and address lines, for example, if Mr. Katrin Müller and Mrs. Walter Schmitt receive mail from you
- Cross- and up-selling opportunities cannot be identified
- Low user acceptance and complaints from employees
- Legal requirements cannot be met
- Lack of planning security: Strategic decisions are only made with great uncertainty
Right up front: Incorrect addresses and poor data quality are a cost factor that companies could simply avoid! In Germany, around 14 million addresses change every year as a result of relocations and around 990,000 as a result of deaths. Many of the total 370,000 marriages and 150,000 divorces each year are associated with name changes. In addition, there are thousands of changes in street names, postal codes and towns each year. Most changes occur at the address - the CRM is not necessarily up-to-date.
Correct postal addresses are of central importance for companies. Only correct addresses ensure the deliverability of mailings, minimize postage and advertising expenses, and are an indispensable prerequisite for identifying duplicates.
The aim is to improve data quality and manage it on an ongoing basis. This is not a one-time task, because almost all data in companies, especially customer data, is subject to constant change. The goal must therefore be to ensure that customer information is constantly available in a consistent, complete and up-to-date form. Nevertheless, companies usually improve their data quality only in phases, for example, because a new project provides an occasion to do so (and corresponding budgets are available). Afterwards, however, the quality usually deteriorates again. This is in the nature of things, because data changes due to new circumstances, such as a change in a mobile phone number or an address.
When we talk about data quality management, we are talking about an approach that ensures the quality of data throughout its entire lifecycle - from its capture, storage and use to its archiving and deletion. The closed-loop approach from Total Quality Management is commonly used here. To begin with, customer data is already checked during data capture using DQ services. Incorrect customer data that cannot be automatically corrected is stored in an intermediate database and a report or alert is sent to the entry point so that it can take corrective action.
This cycle allows customer data to be continuously reviewed as it is entered and processed. If regular reports are written about these processes (for example, via a data quality dashboard), users can measure the performance of the closed loop for data quality management (performance management) and continuously improve the process. The result is almost constant data quality at a high level.
However, data quality does not stop there. Companies are predominantly structured in such a way that DQ management generates an excessive amount of work because data sovereignty usually lies with the departments. As a result, different departments or newly developed business units cannot access all customer data in the company. The data pots do not match. In such constellations, data quality management is limited to separate system silos. These silos contain a lot of customer data, the quality of which could be improved and enriched by merging it with available data throughout the company. But de facto, the existing structures and processes cause high costs due to redundancies.
And what is even more serious: Companies are squandering the great potential that lies in their databases - namely, the opportunity for a unified view of their customers. The reality is sobering, because companies lack an overview and their management can hardly rely on the data as a basis for decisions and measures. Wrong decisions and bad investments can be the expensive consequence. The need for data quality is obvious. However, in order for the success of comprehensive data quality management to actually have an impact on the daily work of employees and the business success of the entire company, master data management is necessary.
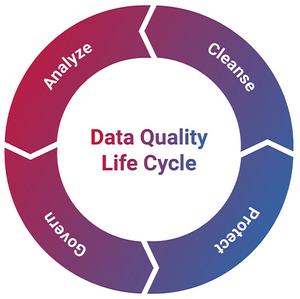
Improving and maintaining data quality requires an end-to-end data quality life cycle. It ensures that the data is given the necessary attention at all times. And that it is always of an appropriate quality. Appropriate in the sense that it is ‘fit for purpose’. With the Data Quality Life Cycle, data quality can be improved and maintained in a closed loop. This includes the following sub-processes:
Analyze
Unknown status of the data quality of all business data
A fundamental prerequisite for the success of data quality initiatives is precise knowledge of the status of the business data in terms of its quality. Completeness, correctness, clarity and consistency are just some of the criteria that must be taken into account when assessing data quality. While customer-related data has been and still is the focus of data quality initiatives, other data domains such as product and material master data, financial data or contract data are increasingly the subject of data governance programmes and organisations within companies.
Cleanse
Initial basic cleansing of business data
Once the current data quality has been determined and targets for quality improvement measures have been set, the task of initial cleansing of all master data (data cleansing) arises. Different master data domains pose their own challenges for the functionality of the tools used in cleansing processes. If standardised address data - specific to defined countries - can be based on fixed specifications of the national postal companies and extensive reference data directories can be used, the considerably more pronounced peculiarities in other data domains require greater individual project- or industry-related efforts to comply with defined business rules in the databases.
Protect
Safeguarding data quality at the point of entry
In interactive applications, for example for customer relationship management, there is a risk of data contamination when new data records are entered, but also when existing data records are changed. This deterioration in quality can be caused by incorrect data entry, multiple storage of the same objects, incorrect utilisation of intended data attributes and much more. In order to counteract the ongoing contamination of the database, data quality assurance by means of a data quality firewall is essential as an integral part of the customer application.
Govern
Continuous monitoring of data quality
In order to implement a data quality strategy effectively and sustainably, the quality of the stored business data must be continuously monitored and reported. Without regular monitoring, there is a risk that the quality level once achieved will be lost over time. Trends should be tracked regularly and alerts should be issued if defined quality thresholds are not met.
Data quality can be compared to rowing against the current. In other words, as soon as you stop rowing, you drift backwards. Data quality deteriorates. This is because data quality is a process that should never end. However, this creeping change is often a gut feeling and therefore not backed up with facts.
You might also be interested in: